By Walt Hickey
Welcome to the Numlock Sunday edition. This week, another podcast edition!
This week, I spoke to MIT Technology Review editor Karen Hao, who frequently appears in Numlock and wrote the bombshell story “How Facebook Got Addicted to Spreading Misinformation.”
The story was a fascinating look inside one of the most important companies on the planet and their struggles around the use of algorithms on their social network. Facebook uses algorithms for far more than just placing advertisements, but has come under scrutiny for the ways that misinformation and extremism have been amplified by the code that makes their website work.
Karen’s story goes inside Facebook’s attempts to address that, and how their focus on rooting out algorithmic bias may ignore other, more important problems related to the algorithms that print them money.
Karen can be found on Twitter, @_Karenhao at MIT Technology Review, and at her newsletter, The Algorithm, that goes out every week on Fridays.
This interview has been condensed and edited.
You wrote this really outstanding story quite recently called, “How Facebook Got Addicted to Spreading Misinformation.” It's a really cool profile of a team within Facebook that works on AI problems, and extensively was working towards an AI solution. But as you get into the piece, it's really complicated. We talk a lot about algorithms. Do you want to go into what algorithms are in the context of Facebook?
What a question start with! In the public conversation when people say that Facebook uses AI, I think most people are thinking, oh, they use AI to target users with ads. And that is 100 percent true, but Facebook is also running thousands of AI algorithms concurrently, not just the ones that they use to target you with ads. They also have facial recognition algorithms that are recognizing your friends in your photos. They also have language translation algorithms, the ones when someone posts something in different language there's that little option to say, translate into English, or whatever language you speak. They also have Newsfeed ranking algorithms which are ordering what you see in Newsfeed. And other recommendation algorithms that are telling you, hey, you might like this page, or you might want to join this group. So, there's just a lot of algorithms that are being used on Facebook's platform in a variety of different ways. But essentially, every single thing that you do on Facebook is somehow supported in part by algorithms.
You wrote they have thousands of models running concurrently, but the thing that you also highlighted, and one reason that this team was thrown together, was that almost none of them have been vetted for bias.
Most of them have not been vetted for bias. In terms of what algorithmic bias is, it's this field of study that has recognized that when algorithms learn from historical data they will often perpetuate the inequities that are present in that historical data. Facebook is currently under a lawsuit from the Housing and Urban Development agency where HUD alleges that Facebook's ad targeting algorithms are showing different people different housing opportunities based on their race, which is illegal. White people more often see houses for sale, whereas minority users more often see houses for rent. And it's because the algorithms are learning from this historical data. Facebook has a team called Responsible AI, but there's also a field of research that's called responsible AI that's all about understanding how do algorithms impact society, and how can we redesign them from the beginning to make sure that they don't have harmful unintended consequences?
And so this team, when they spun up, they were like "none of these algorithms have been audited for bias and that is an unintended consequence that can happen that can legitimately harm people, so we are going to create this team and study this issue." But what's interesting, and what my main critique is in the piece, is there are a lot of harms, unintended harms, that Facebook's algorithms have perpetuated over the years, not just bias. And it's very interesting why they specifically chose to just focus on bias and not other things like misinformation amplification, or polarization exacerbation. Or, the fact that their algorithms have been weaponized by foreign actors to disrupt our democracy. So, that's the main thrust of the piece, is that Facebook has all these algorithms and it's trying, supposedly, to fix them in ways that mitigate their unintended harmful consequences, but it's going about it in a rather narrow minded way.

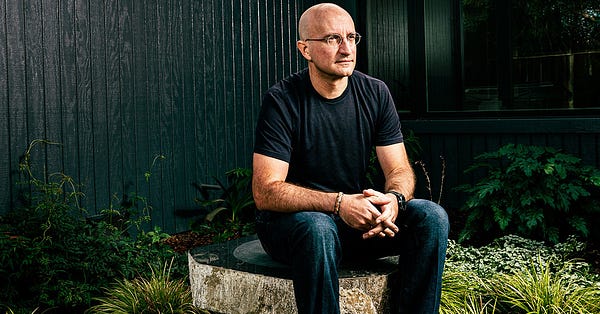
Yeah. It definitely seems to be a situation in which they're trying to address one problem and then alluding to a much larger problem in that. Can you talk a little bit about like, again, one of the issues that they have is that there's this metric that you write about called L6/7. How does their desire for engagement, or more specifically not ever undermining engagement, kneecap some of these efforts?
Facebook used to have this metric called L6/7. I'm actually not sure if it's used anymore, but the same principle holds true, that it has all of these business metrics that are meant to measure engagement on the platform. And that is what it incentivizes its teams to work towards. Now I know for a fact that some of these engagement metrics are the number of likes that users are hitting on the platform, or the number of shares, or the number of comments. Those are all monitored. There was this former engineering manager at Facebook who had actually tweeted about his experience saying that his team was on call, every few days they would get an alert from the Facebook system saying like, comments are down or likes or down, and then his team would then be deployed to figure out what made it go down so that they could fix it. All of these teams are oriented around this particular engagement maximization, which is ultimately driven by Facebook's desire to grow as a company. What's interesting is I realized, through the course of my reporting, that this desire for growth is what dictates what Facebook is willing to do in terms of its efforts around social good. In the case of AI bias, the reason why it is useful for them to be working on AI bias is actually for two reasons.
One is they're already under fire for this legally. They're already being sued by the government. But two, when this responsible AI team was created, it was in the context of big tech being under fire already from the Republican-led government about it allegedly having anti-conservative bias. This was a conversation that began in 2016 as the presidential campaign was ramping up, but then it really picked up its volume in 2018 in the lead up to the midterm elections. About a week after Trump had tweeted #stopthebias in reference to this particular allegation towards big tech, Mark Zuckerberg called a meeting with the head of the responsible AI team and was like, "I need to know what you know about AI bias. And I need to know how we're going to get rid of it in our content moderation algorithms."
And I'm not sure if they explicitly talked about the #stopthebias stuff, but this is the context in which all of these efforts were ramping up. My understanding is Facebook wanted to invest in AI bias so that they could definitively say, "Okay, our algorithms do not have anti-conservative bias when they're moderating content on the platform." And, use that as a way to keep regulation at bay from a Republican-led government.
On the flip side, they didn't pursue many of these other things that you would think would fall under the responsible AI jurisdiction. Like the fact that their algorithms have been shown to amplify misinformation. During a global pandemic, we now understand that that can be life and death. People are getting COVID misinformation, or people were getting election misinformation that then led to the US Capitol riots. They didn't focus on these things because that would require Facebook to fundamentally change the way that it recommends content on the platform, and it would fundamentally require them to move away from an engagement centric model. In other words, it would negatively impact its growth. It would hinder Facebook's growth. And that's what I think is the reason why they didn't do that.


One part that's interesting is Facebook was not instantaneously drawn to AI. When The Facebook was made it didn't involve AI. AI is a solution to another suite of problems that it had in terms of how do you moderate a social network with billions of people, an order of magnitude larger than anyone has ever moderated before, I suppose.
It's interesting. At the time that Facebook started, AI was not really a thing. AI is a very recent thing, it really started to show value for companies in 2014. It's actually really young as a technology, and obviously Facebook started way before 2014. At the time they adopted AI in late 2013, early 2014, because they had this sense that Facebook was scaling really rapidly. There was all of this content on the platform, images, videos, posts, ads, all this stuff. AI, as an academic research field, was just starting to see results in the way that AI could recognize images, and it could potentially one day recognize videos and recognize text and whatever.
And the CTO of the company was like, "Hey, this technology seems like it would be useful for us in general, because we are an information rich company. And AI is on a trajectory to being really good at processing information." But then also what happened at the same time was there were people within the company as well that started realizing that AI was really great at targeting users, at learning users' preferences and then targeting them, whether it was targeting them with ads to or targeting them with groups that they like or pages that they like or targeting them with the posts from the friends that they liked the most.
They very quickly started to realize that AI is great for maximizing engagement on the platform, which was a goal that Facebook had even before they adopted AI, but AI just became a powerful tool for achieving that goal. The fact that AI could help process all of this information on Facebook, and the fact that it could really ramp up user engagement on the platform, collided and Facebook decided we're going to heavily invest in this technology.
There's another stat in your article that really just was fascinating to see laid out. You wrote about how there's 200 traits that Facebook knows about its users, give or take. And a lot of those are estimated. The thing that's interesting about that is I feel like I've told Facebook fairly limited amount of information about myself in the past couple of years. I unambiguously directly told them like, yes, this is my birthday. This is where I live now. This is where I went to college. And then from that, and from their algorithms, and from obviously their myriad cookie tech, they've built this out into a suite of 200 traits that you wrote about. How did AI factor in in that, and how does that lead into this idea of fairness that you get at in the piece?
Yeah, totally. The 200 traits are all about, they're both estimated by AI models, and they're also used to feed AI models. It's dicey territory to ask for race data. Like when you go to a bank, that's part of the reason why they'll never ask you race data because they can't decide banking decisions based on your race. With Facebook that's the same, but they do have a capability to estimate your race by taking a lot of different factors that could highly correlate with certain races. They'll say like, if you are college educated, you like pages about traveling, and you engage a lot with videos of guys playing guitar, and you're male, and you're like within this age, and you live in this town, you are most likely white.
I was about to say, you are a big fan of the band Phish. We're kind of barking up the same tree here, I suspect.
They can do that because they have so much data on all the different things that we've interacted with on the platform. They can estimate things like your political affiliation, if you're engaging with friends' posts that are specifically pro-Bernie or whatever, you are most likely on the left of the political spectrum in the US. Or, they can estimate things like, I don't know, just random interests that you might have. Maybe they figure out that you really like healthy eating, and then they can use that to target you with ads about new vegan subscriptions, whatever it is. They use all of these AI models to figure out these traits. And then those traits are then used to measure how different demographics on Facebook, how different user groups engage with different types of content in aggregate.
The way that this ties to fairness, and then ties to this broader conversation around misinformation? So this Responsible AI team was really working on building these tools to make sure that their algorithms were more fair, and make sure that they won't be accidentally discriminating against users, such as in the HUD lawsuit case, by creating these tools to allow engineers to measure once they've trained up this AI model. Okay, now let's like subdivide these users into different groups that we care about usually based on protected class, based off of these traits that we've estimated about them, and then see whether or not these algorithms impact one group more than another.
So, it allows them a chance to stress test algorithms that are in development against what it hypothetically would do?
Exactly.
Sick.
The issue is that even before this tool existed, the policy team, which sits separately from the Responsible AI team, they were already evoking this idea of anti-conservative bias, and this idea of fairness, to undermine misinformation efforts. So, they would say, "Oh, this AI model that's designed to detect anti-vax information and limit the distribution of anti-vax misinformation, that model, we shouldn't deploy it because we've run some tests and we see that it seems to impact conservative users more than liberal users, and that discriminates against conservatives, so unless you can create this model to make sure it doesn't impact conservative and liberal users differently, then you can't deploy it."
And there was a former researcher that I spoke to who worked on this model, who had those conversations, who was then told to edit the model in a way that basically made the model completely meaningless. This was before the pandemic, but what he said to me was like, this is anti-vax misinformation. If we have been able to deploy that model at full efficacy, then it could be quickly repurposed to anti-vax COVID misinformation. But now we're seeing that there's a lot of vaccine hesitancy around getting these COVID vaccines. And there were things that basically the policy team actively just did in the past that led to this issue not being addressed with full effectiveness.
You talk about this in the piece where instead of fairness being, “we shouldn't have misinformation on the platform, period," it's like, "well, if there's something that could happen that would disproportionately affect one side or the other, we can't do it." Even if one side — I'm making this up — but let's say that liberals were 80 percent of the people who believed in UFOs. And if we had a policy that would roll out a ban on UFO content and it would disproportionately affect liberals, then that would be stymied by this team?
Yes and no. The responsible AI team, what's really interesting is they sent me some documentation of their work. The responsible AI team, they create these tools to help these engineers measure bias in their models. But they also create a lot of educational materials to teach people how to use them. And one of the challenges of doing AI bias work is that fairness can mean many, many different things. You can interpret it to me in many different things.

They have this specific case study about misinformation and political bias, where they're like, if conservatives posted more misinformation than liberals, then fairness does not mean that this model should impact these two groups equally. And similarly, if liberals posted more misinformation than conservatives, fairness means that each piece of content is treated equally. And therefore the model would, by virtue of treating each piece of content equally, impact liberals more than conservatives. But all of these terms are really spongy. Like "fairness," you can interpret it in so many different ways. Then the policy team was like, “we think fairness means that conservatives and liberals cannot be treated differently." And that was what they were using to dismiss, weaken, completely stop a lot of different efforts to try and tamp down misinformation and extremism on the platform.
Where are we at moving forward? It seems like you had alluded to AI being really central to Facebook's policy. And this team, even physically, was close to Mark Zuckerberg's desk. Where are we at moving forward now? Is there a chance that the policy team will lose sway here? Or is there a chance that this is just, it was what it was?
I think what I learned from this piece is that there's just a huge incentive misalignment problem at Facebook. Where as much as they publicly tell us, "we're going to fix these problems, we're going to fix these problems," they don't actually change their business incentives in a way that would allow any of the efforts trying to fix these problems to succeed. So, AI fairness sounds great, but AI fairness in service of business growth can be perverted.
And if the company is unwilling to change those incentive structures, such that truly responsible AI efforts can succeed, then the problems are just going to keep getting worse. The other thing that I realized is we should not be waiting around anymore for Facebook to be doing this stuff because they promised, after the Cambridge Analytica scandal three years ago, that they were going to fix all these things. And the responsible AI team was literally created a couple of weeks after the Cambridge Analytica scandal, as a response to a lot of the allegations that Facebook was facing then about their algorithms harming democracy, harming society.
And in three years, they've just made the problem worse. We went from the Cambridge Analytica Scandal to the US Capitol riots. So, what I learned was, the way that the incentive structures change moving forward will have to come from the outside.
Yeah. Because it is also bigger than just the United States. You alluded in your piece to the genocide in Myanmar. There are much bigger stakes than just elections in a developed democracy.
That was one of the other things that I didn't really spend as much time talking in my piece about, but it is, I think, pretty awful that some of Facebook's misinformation efforts, which impact its global user population, are being filtered based off of US interests. And that's just not in the best interest of the world's population.
Karen, where can people find your work? You write about this stuff all the time and you are the senior editor for AI at MIT Technology Review. So where can folks get ahold of you and find out more about this?
They can follow me on Twitter, @_Karenhao. they can find me on LinkedIn. They could subscribe to MIT Technology Review and once they subscribe they would get access to my subscriber only AI newsletter, The Algorithm, that goes out every week on Fridays.
If you have anything you’d like to see in this Sunday special, shoot me an email. Comment below! Thanks for reading, and thanks so much for supporting Numlock.
Thank you so much for becoming a paid subscriber!
Send links to me on Twitter at @WaltHickey or email me with numbers, tips, or feedback at walt@numlock.news.
Share this post